The exponential growth of generative AI and its technological demands are poised to generate staggering amounts of electronic waste, potentially equating to the weight of 10 billion iPhones per year by 2030.
According to researchers from Cambridge University and the Chinese Academy of Sciences, this prediction highlights the environmental impacts of rapidly advancing AI technology, underscoring the need for sustainable practices in AI hardware production and disposal.
While the researchers don’t propose halting AI’s progress, they aim to bring awareness to the significant environmental implications, particularly the e-waste created from obsolete computing resources, which has yet to receive the same attention as AI’s energy consumption.
A New Wave of E-Waste: AI’s Growing Hardware Needs
The environmental costs of generative AI are twofold: the high energy demands and the substantial waste generated as hardware becomes obsolete. While AI’s energy consumption has sparked conversations on sustainable tech, less attention has been given to the physical lifecycle of AI infrastructure.
With millions of AI servers necessary to support the technology’s rapid growth, e-waste levels could increase by as much as a thousandfold over the coming years.
Researchers model potential scenarios to understand the scale of future AI-generated e-waste. They estimate that e-waste from AI computing needs could rise from 2.6 thousand tons in 2023 to an astounding 0.4–2.5 million tons by 2030.
Read : India Used Digital Technology for People’s Benefit, US Wasted It: Nobel Laureate Paul Romer
This projection, although speculative due to AI’s unpredictable trajectory, is grounded in order-of-magnitude estimations that illustrate the pressing need for eco-conscious practices within the AI industry.
To put these figures into context, the 2.6-kiloton estimate for 2023 excludes infrastructure deployed over the past two years, which is expected to add a significant volume of waste once it reaches end-of-life status. By 2030, the waste generated could indeed reflect an amount equivalent to billions of iPhones.
Read : Punjab Panorama: Exploring the State’s Top Tourist Hotspots
This finding demonstrates the unavoidable link between AI’s evolution and substantial e-waste, underscoring the urgency for environmental interventions.
Strategies to Mitigate AI’s E-Waste Impact
In response to the anticipated e-waste surge, researchers suggest several mitigation strategies. These solutions could potentially reduce e-waste by up to 86%, though actual results depend largely on adoption rates and the scope of implementation.
Options include downcycling servers for reuse, repurposing components like communication modules and power supplies, and improving software to prolong the usefulness of older hardware. By prioritizing efficiency, companies can delay the need for frequent hardware replacements and reduce waste output.
A significant portion of the AI industry’s infrastructure relies on high-powered chips like GPUs, which could either be replaced with more efficient models or repurposed once their initial role ends.
For example, older GPUs could serve in less demanding settings, such as inference tasks in universities, extending their lifecycle and spreading the environmental costs over a longer period. Companies could also emphasize updating to high-efficiency chips as soon as possible to avoid using multiple outdated models that generate more waste.
However, while these strategies could mitigate some of AI’s environmental impact, their success largely depends on industry-wide commitment.
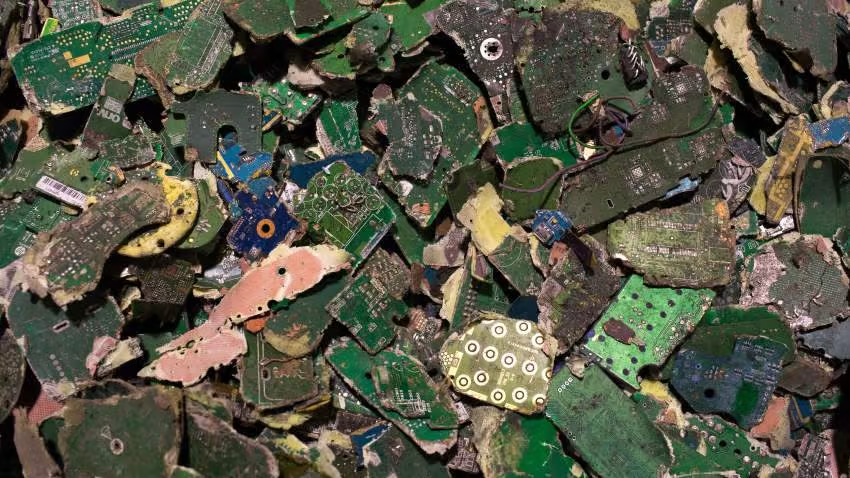
The potential benefits of adopting these solutions are considerable. For instance, by embracing downcycling, organizations could achieve both cost savings and environmental benefits. Components like hard drives and power supplies can also be repurposed, offsetting the need for new resources and reducing waste.
On the software side, optimizing code for older chips could delay the need for hardware upgrades, further reducing waste. However, without broad adoption, these potential reductions remain theoretical, highlighting the importance of industry leaders in adopting sustainable practices.
The Environmental Choice: How Industry Decisions Will Shape E-Waste Levels
The disparity between the low and high estimates for e-waste—spanning from hundreds of thousands to millions of tons—points to the choices the tech industry faces in shaping AI’s environmental footprint.
If companies maximize hardware reuse and opt for energy-efficient models, they can help curb the e-waste impact. On the other hand, a “business-as-usual” approach, in which older hardware is frequently replaced without recycling or repurposing, could escalate waste to unprecedented levels.
The study underscores that achieving lower e-waste levels will require concerted action and commitment from tech companies. For instance, reusing a significant percentage of AI servers rather than discarding them would contribute to a lower waste outcome.
Even if only 10% of AI hardware finds a second life, the environmental benefits are noteworthy, though the study estimates much greater gains with higher participation.
To reach a sustainable trajectory, it’s vital that companies consider the entire lifecycle of their computing infrastructure, from production and operation to disposal.
By extending the functional life of each server or chip, companies can mitigate e-waste while also conserving the natural resources required to manufacture new equipment. Ultimately, these choices will define AI’s environmental legacy.
let’s enjoy few years on earth with peace and happiness….✍🏼🙏